Implementation Research is a Team Sport: Make Sure You have a Statistician on Your Side!
The role of the statistician in implementation research (IR) is commonly restricted to the conduct of data analysis at the end of a study. But this limited perception may be causing unnecessary challenges and delays to the research process. We spoke with Augusto Norte from A Consultants Statistical Services about the benefits of including a statistician as part of the project team, and about his experiences supporting a Global Alliance for Improved Nutrition (GAIN) project in Mozambique.
Why is it important that a statistician is part of the project team?
I truly believe that the statistical consultant is a strategic partner for the overall success of a project. At first it may seem unnecessary to include a statistician from the beginning of a project, and although their input during these stages may be minimal, it can lead to big gains in efficiency later on.
For example, a statistician can review the study protocol and highlight the potential risks of omitting specific information, or limitations associated with analyzing data captured in a certain way. These insights can help the project team make proactive revisions and timely decisions during the design phase. Similarly, it is essential to inform the statistical consultant of any proposed changes to the protocol that may arise during the project implementation, so that they can provide input on its potential impact on data management and analysis. In this way, future problems can be avoided.
From my point of view, the statistical consultant is there to provide valuable support to the project team and it is essential that they are deemed part of this team from its inception.
Tell us about the GAIN project you were involved with in Mozambique.
Micronutrient powders (MNP) are recommended by the World Health Organization as an effective intervention to address anemia in young children.
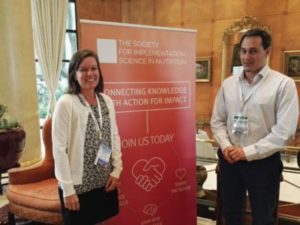
August Norte, Statistical Consultant with Alison Tumilowicz from GAIN
GAIN implemented a MNP delivery model using a voucher distribution system in two districts of Sofala Province in Mozambique.
A formative evaluation of this model included the examination of program outcomes using routine monitoring data and a population-based cross-sectional survey among caregivers of children 6-23 months.
I had a 7-month statistical consulting services contract to complete functional and coding revisions of questionnaires, design and testing of data entry masks, data cleaning, data analysis, preparation of results tables, a training workshop in Maputo, and to provide technical support to GAIN’s local data collection agency for both the process and impact evaluations of the MNP project.
Can you describe some of the challenges you faced when conducting the statistical analysis for this project?
In every project, knowledge evolves throughout the research process. In the case of the survey questionnaire, the version administered at end-line was different to the questionnaire administered at baseline. These changes included the addition of new questions, omission of redundant questions, and reformulation of other items to capture the data in a better way.
To perform a comparative analysis between the districts surveyed in the baseline and in the end-line survey, it was necessary to merge the two databases. In cases where there was no change, this was not a problem. But for questions where changes had been made it was necessary to analyze the differences one by one and make decisions on how to merge on a case by case basis. Thankfully, the changes could be saved in most cases, with the exception of questions that were removed and those that were added.
Another challenge when merging the databases and performing comparative analysis, was that the question codes in the baseline questionnaire did not match the codes of the same questions in the end-line version. Nor did the names of the variables in the database match. This was mainly because the statistical consultancy provider for the baseline survey was not the same as for the end-line survey. This lack of consistency meant that the time required to prepare the data for analysis was extended.
What were some of the key lessons learned from your experience consulting on this project?
- Open and timely communication
Data collection, entry and cleaning took longer than expected which delayed when the datasets were ready for analysis. This highlights the importance of all providers and stakeholders remaining in close communication with the project manager. Thus, if a process is taking longer than anticipated, decisions can be made to reduce or accommodate the delay.
- Use of technology
The questionnaires administered in the project were paper-based. During the data cleaning phase, we identified many inconsistencies in the database which needed verification by sending e-mails to those with the original documents. However, the answers received created new inconsistencies and additional questions. Subsequently, GAIN consultants were asked to obtain the original surveys and verify the data themselves.
This scenario is not uncommon when data is abstracted from paper-based surveys and entered into a database. Even with checks built into the database, human error frequently occurs. Conducting an electronic survey (with tablets or mobiles) negates the need for additional data entry and reduces these errors. Of course, the interviewer may still make a mistake when entering information but as they receive a warning message at the time of administering the survey their response can be corrected immediately, which drastically reduces instances in which inconsistencies may be generated.
- Be prepared for the unexpected
My previous responses probably show that even with careful planning challenges still occur. Another unexpected complication was related to the sample. Probability-proportional-to-size sampling methodology was employed to select the sample. However, due to unforeseen challenges, the project team had to make the decision to treat it as a convenience sample rather than representative of the project area, thus changing the evaluation design.
More About Augusto Norte:
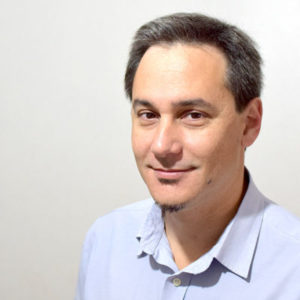
Augusto Norte
Augusto is an international statistical consultant and university professor specialized in Statistical Analysis and Project Management for Research Studies. In December 2015 he founded A-Consultants, a statistical consulting team providing statistical consultancy services, training and statistical coaching.
In his more than 15 years of professional experience he has conducted statistical analysis providing support in survey planning, data collection, data cleaning, statistical data analysis and survey reports. Augusto is an expert in the statistical package IBM SPSS Statistics. In academia, he has taught multiple courses on Methodological and Statistical Data Analysis, he has provided statistical support to researchers from the Argentinean National Council for Scientific and Technical Research (CONICET) and has also supervised PhD candidates in various disciplines.
He is a University Professor and teaches Statistics and Probability at Facultad de Ciencias Exactas y Naturales at Universidad Nacional de Cuyo, Mendoza, Argentina. In the last 3 years he has worked with professionals from Global Alliance for Improved Nutrition (GAIN), CDC from USA and GroundWork Health in different projects from African countries such as Mozambique, Ethiopia, Ivory Coast and Burkina Faso. In 2016 he led a workshop in Maputo, Mozambique for MoH, GAIN, INE and local consultants.
In the private sphere, he has worked for multinational companies and small businesses leading projects, carrying out implementation of continuous improvement processes such as Lean Manufacturing and conducting data analysis projects.